Machine learning deployed to fast-track industrial optimization process
Joint research project between science and industry -
An interdisciplinary team of researchers at the Max Planck Institute for Intelligent Systems, the Max Planck Institute for Solid State Research, the Technical University of Munich, and Robert Bosch GmbH deploy Bayesian Machine Learning methods to fast-track the optimization process of coating copper used in microchips. By doing so, the optimization process can be speeded up fifteenfold compared to taking a conventional optimization approach.
Stuttgart/Renningen/Reutlingen – Microchips are a building component of practically every electrical product. One metal very common in the production of these chips is copper. This soft metal is essential in the manufacturing of microchip-based products, as it offers both electrical and thermal conductivity. However, for copper to unleash its full potential, its corrosion must be avoided. In the production process of microchips, it is therefore necessary to coat the copper with a thin layer of ceramic material: aluminum oxide. This encapsulating process is called Atomic Layer Deposition or ALD in short.
The optimization of the coating process is tedious and costly
The coating of copper is not a dip-and-go process. It takes a lot of engineering expertise, many hours of manual labor, and tedious trials to find the optimum deposition parameters for the layer to be defect-free (i.e., without any holes, dents or gaps) and to be as thin as possible. Firstly, because there is little space in microchips often the size of a dust mite. Secondly, aluminum oxide is costly: scaled up to the production of thousands if not millions of microchips, even a few nanometers more width of the coating layer puts a dent in the margin. And thirdly, gaps and dents are the back door for corrosion to find its way in after all.
An interdisciplinary team of researchers at the Max Planck Institute for Intelligent Systems, the Max Planck Institute for Solid State Research, the Technical University of Munich, and Robert Bosch GmbH – an industrial partner in the Cyber Valley research network – has found a way to minimize defects in the layer, to fast-track the optimization process and to reduce the costs resulting from coating copper used in microchips. They deployed Bayesian Machine Learning methods and found that the optimization process can be speeded up fifteenfold compared to taking a conventional optimization approach.
Bayesian optimization
“Imperfections in the coating layer can be reduced by having a thicker coating film, however then it costs more and the layer takes up more space. Many iterations are necessary for engineers to find the ideal coating with little to few defects. On an industrial scale, it takes even more time, weeks maybe months, and hence it costs thousands of euros for each iteration. Therefore, reducing the optimization process from 30 or 40 steps to only two or three through Bayesian optimization is quite an achievement,” says Gül Dogan, a Ph.D. student both in the Modern Magnetic Systems Department at the Max-Planck-Institute for Intelligent Systems (MPI-IS) and with Robert Bosch GmbH. She is the first author of Bayesian Machine Learning for Efficient Minimization of Defects in ALD Passivation Layers, recently published in the journal Applied Materials and Interfaces.
“We use machine learning to perform better than exhaustive conventional search and optimization methods. Our algorithm collaborates with the experts and learns from their experience – with success: We have virtually no film-porosity and hence significantly reduce the corrosion,” Sinan Demir adds, who is a Ph.D. student in the Physical Intelligence Department at MPI-IS, led by Prof. Metin Sitti.
Several parameters determine how good the coating outcome is: how long the copper surface is pretreated using Ar/H2 plasma to clean the material, the deposition temperature above 200 °C, and a 60 ms pulse time applying the aluminum oxide. All parameters and their interplay influence the corrosion resistance. The algorithm was capable of finding the optimal parameter set in only a few iterations.
Learnings can be transferred
“Let’s assume the system performance is defined by this orange function seen in Figure 1. Just assume that our parameters affect the production process according to this function. We start with no knowledge at all. As we collect new data points – the blue markers in the lower image – we update the prediction about the system and based on this prediction, Bayesian optimization suggests a new parameter set to be tested, which can improve the performance of the system. Then, we do the experiment by using this new parameter set. We continue testing and choosing new parameters sets until we reach our final goal or design criteria,” Demir continues.
The coating of copper is one optimization challenge. In materials science, there are many more. “This is the advantage of Bayesian Machine Learning methods. The learnings you gain from one optimization process can be transferred to different materials and processes in all areas of materials science. You don’t start at zero, you can scale it up,” Demir concludes.
Wissenschaftlicher Ansprechpartner:
Gül Dogan guel.dogan@de.bosch.com
Sinan Demir demir@is.mpg.de
Originalpublikation:
https://pubs.acs.org/doi/abs/10.1021/acsami.1c14586
Weitere Informationen:
https://is.mpg.de/en/news/machine-learning-deployed-to-fast-track-industrial-optimization-process
Die semantisch ähnlichsten Pressemitteilungen im idw
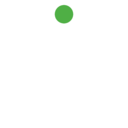