Fraunhofer HHI, TU Berlin and BIFOLD present new method for Explainable AI
Artificial Intelligence is already in widespread use. Yet it remains difficult to understand how an AI system reaches its decisions. Scientists at the Fraunhofer Heinrich-Hertz-Institut (HHI) and the Berlin Institute for the Foundations of Learning and Data (BIFOLD) at TU Berlin have collaborated for many years to make AI explainable. Now the scientists around Prof. Thomas Wiegand (Fraunhofer HHI, BIFOLD), Prof. Wojciech Samek (Fraunhofer HHI, BIFOLD) and Dr. Sebastian Lapuschkin (Fraunhofer HHI) have achieved another milestone.
In their paper "From attribution maps to human-understandable explanations through Concept Relevance Propagation", the researchers present Concept Relevance Propagation (CRP), a new method for explainable AI that can explain individual AI decisions as concepts understandable to humans. The paper has now been published in the international journal Nature Machine Intelligence.
AI systems are largely black boxes: It is usually not comprehensible to humans how an AI arrives at a certain decision. CRP is a state-of-the-art explanatory method for deep neural networks that complements and deepens existing explanatory models. In doing so, CRP reveals not only the characteristics of the input that are relevant to the decision made, but also the concepts the AI used, the location where they are represented in the input, and which parts of the neural network are responsible for them. Thus, CRP is able to explain individual decisions made by an AI using concepts that are understandable to humans. As a result, this research sets an entirely new standard for the evaluation of and interaction with AI.
For the first time, this approach to explainability takes a look at the entire prediction process of an AI – all the way from input to output. In recent years, the research team has already developed various methods for using so-called heat maps to explain how AI algorithms reach their decisions. The heat maps highlight specific areas in an image that are particularly relevant to the decision made. This method has become known as Layer-wise Relevance Propagation (LRP). The importance of this type of explainability is enormous, as it allows us to understand whether an AI is actually making decisions based on sound reasoning or whether it has merely learned shortcut strategies and is thus cheating.
The new CRP method draws on Layer-wise Relevance Propagation. "AI image recognition is a good example of this," says Prof. Wojciech Samek, head of the "Artificial Intelligence" department at Fraunhofer HHI, professor of Machine Learning and Communications at TU Berlin, and BIFOLD Fellow. "On the input level, CRP labels which pixels within an image are most relevant for the AI decision process. This is an important step in understanding an AI's decisions, but it doesn't explain the underlying concept of why the AI considers those exact pixels." For comparison, when humans see a black-and-white striped surface, they don't automatically recognize a zebra. To do so, they also need information such as four legs, hooves, tail, etc. Ultimately, they combine the information of the pixels (black and white) with the concept of animal.
"CRP transfers the explanation from the input space, where the image with all its pixels is located, to the semantically enriched concept space formed by higher layers of the neural network," states Dr. Sebastian Lapuschkin, head of the research group "Explainable Artificial Intelligence" at Fraunhofer HHI, elaborating on the new method. "CRP is the next step in AI explainability and offers entirely new possibilities in terms of investigating, testing and improving the functionality of AI models. We are already very excited to apply our new method to large language models like ChatGPT."
Wissenschaftlicher Ansprechpartner:
Prof. Wojciech Samek
Email: wojciech.samek@hhi.fraunhofer.de
Phone: +49 30 31002-417
Weitere Informationen:
https://doi.org/10.1038/s42256-023-00711-8
Die semantisch ähnlichsten Pressemitteilungen im idw
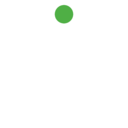