How to open the black boxes of AI / ISM business information scientist Marcus Becker calls for transparency algorithms
Human investment advisors can be liable for damages - in principle; this also applies to automated advisors. However, who is behind it? Prof. Dr. Marcus Becker uses this example to address a central question that is becoming increasingly urgent with the use of artificial intelligence (AI). Even more so as the conspicuous lack of transparency in AI processes suggests that this is almost methodical in nature. The expert from the International School of Management (ISM) shows where practical steps can be taken to open up AI black boxes.
Marcus Becker has been working with machine learning methods for more than five years. When analyzing the learning methods of AI, the mathematician is most fascinated by the application of his discipline: "Machine learning algorithms combine several areas of mathematics in an extremely elegant way. What surprises me is how easy it is nowadays to generate complex programming codes quickly - with Chat GPT even without mastering the programming language."
The results of AI fascinate both experts and the public, who are optimistic for this method, which is used to select existing facts and combine them in an amazingly useful way according to the principle of probability. This is where Becker comes in. If you want to understand these complex models, serious questions arise: "Artificial neural networks are so-called black box algorithms. This means that we cannot determine in advance exactly how the model will deal with given input information."
A blessing and a curse at the same time?
Becker therefore suggests finding out what criteria the algorithm uses to identify information as "best or suitable facts". Especially as there are quasi self-reinforcing algorithms: "This happens through continuous interaction with their environment. The assessment of the probability of how algorithms can continue to interact with their environment in the future naturally plays a decisive role here."
As a stochastics expert, Becker is delighted at how well the application of probability theory works in practice. However, as a researcher, he also feels committed to the search for truth. He is primarily concerned with the problem of verifiability. Artificially generated predictions by AI should not be accepted unchecked, especially when it comes to fundamental decisions. Documented proof of the accuracy of the AI results would be important: "Only then can black box algorithms be used sensibly as a lever for human decision-making competence."
Becker examines the question specifically in the case of automated investment advice based on AI methods. The liability and the question of culpability, for example, remain unresolved. Becker points out that German liability law assumes a breach of duty that leads to damage. However, how can this be clarified when even developers of AI methods are often no longer able to find the error: "This causality problem causes major problems. One of them is the provability of breaches of duty. This raises the question of who bears the burden of proof in such constellations. Since AI systems are not considered to be an independent legal entity, the current legal situation is therefore based on the misconduct of the user who uses the black box algorithm, in accordance with Section 280 (1) of the German Civil Code." In other words, it is the user's own fault if they trust black box algorithms.
When knowledge bubbles solidify
Legislators or regulatory bodies are needed. Becker welcomes the EU's recent push for AI regulation, the so-called "EU AI Act", but remains skeptical: "The regulatory push for AI liability points in the right direction. A uniform AI regulation is to be welcomed. However, it remains to be seen whether it will reduce the risks associated with the use of AI, for example because the question of independent legal personality remains unresolved.
The mathematics professor also points out where society is heading if it surrenders to black box algorithms without questioning them. Becker also points out that a technology that continuously accesses and compiles what we already know and have learned "will have an impact on our level of knowledge in general if our level of information no longer expands. We will then find ourselves in a knowledge bubble. This is likely to at least slow down progress."
Explainable AI
There is an obvious way out, as Marcus Becker adds - to retain the upper hand over the algorithms: "I call for the use of transparency algorithms, so-called Explainable AI or XAI for short." This would open up the black box of AI methods. Because: "There are a large number of explanatory models (such as LIME and SHAP) that are virtually universally applicable. However, companies hardly use them, partly because law does not prescribe them. However, they would increase user confidence.
AI-controlled financial planning tools, so-called "robo advisors", could in principle be brought into line with data protection regulations. Nevertheless: "Without additional explanation systems, the decisions of the black boxes cannot be interpreted and checked for their economic validity".
Wissenschaftlicher Ansprechpartner:
Prof. Dr. Marcus Becker
Weitere Informationen:
http://presse@ism.de
Die semantisch ähnlichsten Pressemitteilungen im idw
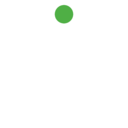