Why is mathematics indispensable for artificial intelligence?
While artificial intelligence (AI) is perceived today as a subfield of computer science, many people are unaware that it is a highly interdisciplinary field that benefits massively from ideas from mathematics, as five mathematical societies have now jointly declared.
Five major mathematical societies have jointly agreed on a statement on the importance of mathematics for artificial intelligence (AI).
„A person who refuses to deal with arithmetic is doomed to talk nonsense". This sentence comes from John McCarthy, Professor of Artificial Intelligence and one of its founding fathers. While AI is now perceived as a subfield of computer science, many people do not realize that it is a highly interdisciplinary field that benefits massively from ideas from mathematics. Mathematics helps to increase the safety and efficiency of AI systems.
Four examples:
- Generative AI, which generates amazingly realistic images from simple text input, uses sophisticated mathematical concepts such as diffusion models based on stochastic differential equations.
- Modern AI systems are usually based on complex neural networks. It is now known that neural networks can be unstable: The smallest disturbances in the input data (e.g. so-called adversarial attacks) can lead to massive errors in the results. In practical applications such as autonomous driving or medical diagnostics, this poses a considerable safety risk. Mathematics is researching how such instabilities can be controlled by improving the design of neural networks.
- Neural networks in AI systems depend on millions and millions of parameters. This makes them opaque to us humans. The aim of mathematics is to develop more compact models that require fewer parameters for the same performance, are more transparent and therefore more explainable and also enable performance guarantees in critical applications.
- In order for neural networks to deliver the required performance, their parameters have to be trained at great computational expense. This consumes enormous energy resources. Forecasts assume that future AI systems worldwide will consume the electricity requirements of entire countries such as the Netherlands, Sweden or Argentina. By developing modern optimization methods, mathematics is making the training of neural networks more efficient and resource-saving.
Although it is often claimed that AI is a black box, all elements of AI can actually be explained with mathematical precision. This includes the statistical understanding of the data, the training objectives, the training methods and the network architectures. All of this allows us to understand the success of neural networks, for example. A sound mathematical education makes it possible to model training objectives in such a way that important aspects, such as security considerations, can be taken into account during training. In addition, abstract mathematical thinking allows solutions from certain application domains to be efficiently transferred to other areas. "All of this makes it clear that mathematics plays a key role in the development and understanding of AI," say the authors of the statement. The statement was signed by the presidents of the following professional associations:
DMV: German Mathematical Society
GAMM: Society for Applied Mathematics and Mechanics
GIP: Society for Inverse Problems
GOR: Society for Operations Research
Committee for Mathematical Modeling, Simulation and Optimization
You can also find the entire statement at https://www.mathematik.de/dmv-blog
Originalpublikation:
https://www.mathematik.de/dmv-blog
Die semantisch ähnlichsten Pressemitteilungen im idw
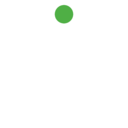