New AI model TabPFN enables faster and more accurate predictions on small tabular data sets
• A team led by Frank Hutter, Professor of Machine Learning at the University of Freiburg, has developed a new method that facilitates and improves predictions of tabular data, especially for small data sets with fewer than 10,000 data points.
• The new AI model TabPFN is trained on synthetically generated data before it is used and thus learns to evaluate possible causal relationships and use them for predictions.
• Hutter: “Many disciplines can benefit from this method and thus also recognise important relationships faster and more reliably than before, even with limited data.”
Filling gaps in data sets or identifying outliers – that’s the domain of the machine learning algorithm TabPFN, developed by a team led by Prof. Dr. Frank Hutter from the University of Freiburg. This artificial intelligence (AI) uses learning methods inspired by large language models. TabPFN learns causal relationships from synthetic data and is therefore more likely to make correct predictions than the standard algorithms that have been used up to now. The results were published in the journal Nature. In addition to the University of Freiburg, the University Medical Center Freiburg, the Charité – Berlin University Medicine, the Freiburg startup PriorLabs and the ELLIS Institute Tübingen were involved.
Data sets, whether they are on the effects of certain medications or particle paths in accelerators at CERN, are rarely complete or error-free. Therefore, an important part of scientific data analysis is to recognise outliers as such or to predict meaningful estimates for missing values. Existing algorithms, such as XGBoost, work well with large data sets, but are often unreliable with smaller data volumes.
With the TabPFN model, Hutter and his team solve this problem by training the algorithm on artificially created data sets that are modelled on real scenarios. To do this, the scientists create data tables in which the entries in the individual table columns are causally linked. TabPFN was trained with 100 million such synthetic data sets. This training teaches the model to evaluate various possible causal relationships and use them for its predictions.
The model especially outperforms other algorithms for small tables with fewer than 10,000 rows, many outliers or a large number of missing values. For example, TabPFN requires only 50% of the data to achieve the same accuracy as the previously best model. In addition, TabPFN is more efficient than previous algorithms at handling new types of data. Instead of starting a new learning process for each data set, the model can be adapted to similar data sets. This process is similar to the adaptation of language models with open weights like Llama, developed by Meta. The model also makes it possible to derive the probability density from a data set and to generate new data with similar properties from it.
‘The ability to use TabPFN to reliably and quickly calculate predictions from tabular data is beneficial for many disciplines, from biomedicine to economics and physics,’ says Hutter. ’TabPFN delivers better results faster and, because it requires few resources and data, is ideal for small companies and teams.’ The code and instructions on how to use it can be found here. In the next step, the researchers will further develop the AI so that it can make the best possible predictions even with larger data sets.
• Original publication: N. Hollmann, S. Müller, L. Purucker, A. Krishnakumar, M. Körfer, Shi Bin Hoo, R. T. Schirrmeister, F. Hutter: Accurate Predictions on Small Data with a Tabular Foundation Model. Nature, 2025. URL: https://www.nature.com/articles/s41586-024-08328-6 . DOI: 10.1038/s41586-024-08328-6
• Noah Hollmann is a research assistant at the Chair of Machine Learning at the University of Freiburg, a student at Charité – Berlin University Medicine and the Berlin Institute of Health at Charité (BIH), and a cofounder of PriorLabs. Samuel Müller and Lennart Purucker are doing their doctorates under Prof. Dr Frank Hutter, Arjun Krishnakumar is a research associate at Hutter's professorship. Max Körfer was also a doctoral student under Hutter, Shi Bin Hoo works as a student assistant at the Chair of Machine Learning. Dr Robin Tibor Schirrmeister is a research associate at the Department of Diagnostic and Interventional Radiology at the Medical Center – University of Freiburg. Prof. Dr Frank Hutter heads a research group at the ELLIS Institute in Tübingen in addition to his professorship at the University of Freiburg, and is also a cofounder of PriorLabs.
• The research was funded by the state of Baden-Württemberg and the German Research Foundation (DFG) through the high-performance computer NEMO (INST 39/963-1 FUGG); by the DFG under project number 417962 828 and as part of the Collaborative Research Centre SmallData, project number 499552394; and by the European Union with the ERC Consolidator Grant DeepLearning 2.0, No. 101045765.
Wissenschaftlicher Ansprechpartner:
Prof. Dr. Frank Hutter
fh@cs.uni-freiburg.de
Originalpublikation:
https://www.nature.com/articles/s41586-024-08328-6
Weitere Informationen:
https://uni-freiburg.de/en/new-ai-model-tabpfn-enables-faster-and-more-accurate-predictions-on-small-tabular-data-sets/
Die semantisch ähnlichsten Pressemitteilungen im idw
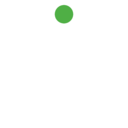