Ethics in Artificial Intelligence: Do AI Systems Provide Accurate and Fair Answers?
Hof, Germany – The rapid development of artificial intelligence (AI) brings not only technological advancements but also complex ethical questions. Particularly in the case of generative AI, such as text and image generation models, the issue of biased outcomes has come under scrutiny. Professors Dr. René Peinl, Marc Lehmann, and Dr. Andreas Wagener from the Institute for Information Systems (iisys) at Hof University of Applied Sciences have analyzed this issue and arrived at intriguing findings.
Bias in AI refers to the tendency of models to produce outcomes that are skewed or influenced by human prejudices. “These distortions often result from the data used to train the models and the way the algorithms process that data. Studies often assume that what constitutes a ‘correct’ or ‘unbiased’ response is clearly defined,” explains Prof. Dr. René Peinl. However, societal reality shows that such definitions are frequently highly contested.
Who Decides What is ‘Correct’?
In practice, there is no consensus on what constitutes a "correct" or "fair" answer. Topics like gender-inclusive language, anthropogenic climate change, or LGBTQ+ equality are often hotly debated within society. “When an AI model appears to give a biased response to a question, it raises the question of whether this is truly an expression of bias—or simply the statistically most likely answer,” elaborates Prof. Dr. Andreas Wagener.
For example, a generated image of a “Bavarian man” often depicts a man in lederhosen holding a beer stein. While this depiction may seem stereotypical, it reflects cultural symbolism that conveys a clear association for many. A man in a suit or tracksuit would not evoke the same cultural context.
Technical Limitations
Many seemingly biased outcomes stem from model quality and the inputs themselves. “AI models often have to make decisions when inputs are vague or underspecified. For instance, a generic prompt like ‘cow’ might lead a model to generate mostly images of cows in fields or barns—an example of bias, though arguably a desirable one,” explains Marc Lehmann.
Additionally, unclear prompts force models to select probable interpretations. Improving model outcomes therefore requires more precise inputs and a detailed understanding of statistical distributions.
Potential Solutions
The researchers at Hof University have explored various approaches to minimizing bias but found no universal solution. Divisions within Western societies further complicate the task of designing models that achieve broad acceptance. In some cases, statistical distributions can serve as a guide. “For example, image generators should represent men and women equally in gender-neutral professions to avoid perpetuating historical discrimination,” suggests Prof. Dr. René Peinl.
Accounting for Minorities
In other cases, however, equal representation is not practical. For example, around 2% of Germany's population identifies as homosexual. A model that generates one in four images of “happy couples” as homosexual would significantly overrepresent statistical reality. Instead, an AI model should accurately respond to explicit prompts like “gay couple” and generate corresponding images.
Country-Specific Defaults: A Pragmatic Compromise?
Another suggestion from the researchers involves introducing country-specific defaults. For instance, a prompt for “man” could generate an Asian man in China, a Black man in Nigeria, and a Caucasian man in Germany. These adjustments would account for cultural and demographic differences without being discriminatory.
Striking a Balance Between Precision and Neutrality
The research highlights the immense challenge of developing unbiased AI models. There are no easy answers, as many problems stem from societal disagreements. A potential solution is to design models that accurately reflect clear inputs while considering country-specific contexts. However, even these approaches require ongoing discussion and adaptation to meet ethical and technical standards.
Wissenschaftlicher Ansprechpartner:
Prof. Dr. René Peinl
+49 9281 409 - 4820
rene.peinl@hof-university.de
Die semantisch ähnlichsten Pressemitteilungen im idw
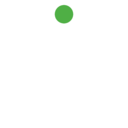